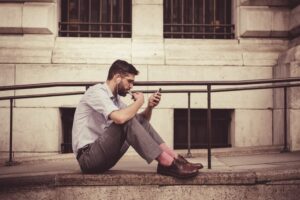
What is Digital Phenotyping?
Digital phenotyping provides an objective way to gather data through digital devices such as Smartphones, which can improve research and clinical practice by avoiding the pitfalls of limited data or self-reporting as the basis of diagnosis (Insel, 2018). This new approach to data collection, which was first defined less than five years ago, employs sensors that track activity and location (Jain, Powers, Hawkins, & Brownstein, 2015). It can also utilize voice and speech recognition innovations as well as human-computer interaction, which can provide critical information on someone’s cognitive and affective states.
Conventional psychological tests evaluate the same types of phenomena that we can now test by looking at how people interact with their Smartphones. Smartphones are even more valuable for these types of assessments when you consider that they are conducive to the gathering of information on a continuous basis and through direct measurement in a non-laboratory setting. Also important is that psychologists and psychiatrists have looked more and more to behavior as indicative of psychiatric state (Insel, 2017) and behavioral data is best collected on an ongoing basis outside the clinic.
By allowing people to stay in their native environments while data collection occurs, it is easier to gain insights into people’s natural behaviors and underlying states (Insel, 2018). For instance, a person reporting to another person about their depressive symptoms and at a time when they are possibly not experiencing those symptoms may provide a clinician with a significantly different picture than would be provided by direct measurements occurring during the depressive episodes. Digital phenotyping may offer a way to determine or monitor the severity of one’s depression (Jacobson, Weingarden, & Wilhelm, 2019).
How is Digital Phenotyping Helpful?
This new digital phenotyping approach is being investigated in different areas of psychological and medical research, and the research is providing hope that digital phenotyping will prove valuable in areas like psychiatry and neurology (Raballo, 2018). For instance, for high risk mental illness groups, such as those in the postpartum state or who are victims of trauma, the information that is gathered through digital phenotyping may allow clinicians to identify a status shift from at-risk to in-need of intervention (Insel, 2018). Psychiatrists may be able to monitor, for instance, behavior consistent with suicidal thoughts or schizophrenia relapse (Kleiman et al., 2018; Marsch, 2018).
Importantly, experts predict that digital phenotyping may become an increasingly useful way for clinicians to meaningfully engage with their patients over an extended period of time (Torous, 2019). Such engagement could provide critical help for at-risk individuals.
Research on the utility of digital phenotyping for the clinical management of those with amyotrophic lateral sclerosis (ALS), a neurodegenerative disease, has shown that this strategy is not only feasible but also can provide important information to clinicians on the progression of the disease (Berry et al., 2019). Specifically, results on tests related to ALS that are conducted through Smartphones appear to correlate highly with results gathered in the clinic, suggesting that digital phenotyping can provide an efficient way to remotely monitor ALS status.
The value of digital phenotyping will likely not be restricted to evaluating the status of the brain. New research has shown that by providing data related to sleep patterns, this approach may help in the identification of cardiovascular disease risk and biological aging (Teo et al., 2019).
Data Types
Data that are gathered through digital phenotyping are categorized into two types: active data and passive data. As the term suggests, active data requires that users actively input data, such as through surveys or the recording of voice or audio information.
Passive data, on the other hand, can be collected through sensors and data on phone usage without the user having to be actively engaged. Passive data may tap into location information, through things like GPS and through this type of system may also be able to provide information on users’ movement and motor activity. Through Wifi routers and Bluetooth devices, data can also be collected on phone usage patterns and the substance of that usage, including the details of social interactions.
Conclusion
Digital phenotyping and its applications are still being developed and optimized. Interdisciplinary teams are working hard to not only understand the potential of digital phenotyping but also to ensure that technologies are built that are conducive to the best use of digital phenotyping and that clinicians and patients who employ digital phenotyping understand how best to use the approach to achieve desired outcomes (Vaidyam, Halamka, & Torous, 2019).
References
Berry, J. D., Paganoni, S., Carlson, K., Burke, K., Weber, H., Staples, P., … Onnela, J. P. (2019). Design and results of a smartphone-based digital phenotyping study to quantify ALS progression. Annals of Clinical and Translational Neurology, 6(5), 873–881. https://doi.org/10.1002/acn3.770
Insel, T. R. (2017). Digital Phenotyping: Technology for a New Science of Behavior. JAMA, 318(13), 1215–1216. https://doi.org/10.1001/jama.2017.11295
Insel, T. R. (2018, October). Digital phenotyping: a global tool for psychiatry. World Psychiatry : Official Journal of the World Psychiatric Association (WPA). Italy. https://doi.org/10.1002/wps.20550
Jacobson, N. C., Weingarden, H., & Wilhelm, S. (2019). Using Digital Phenotyping to Accurately Detect Depression Severity. The Journal of Nervous and Mental Disease, 207(10), 893–896. https://doi.org/10.1097/NMD.0000000000001042
Jain, S. H., Powers, B. W., Hawkins, J. B., & Brownstein, J. S. (2015). The digital phenotype. Nature Biotechnology, 33(5), 462–463. https://doi.org/10.1038/nbt.3223
Kleiman, E. M., Turner, B. J., Fedor, S., Beale, E. E., Picard, R. W., Huffman, J. C., & Nock, M. K. (2018). Digital phenotyping of suicidal thoughts. Depression and Anxiety, 35(7), 601–608. https://doi.org/10.1002/da.22730
Marsch, L. A. (2018). Opportunities and needs in digital phenotyping. Neuropsychopharmacology : Official Publication of the American College of Neuropsychopharmacology, 43(8), 1637–1638. https://doi.org/10.1038/s41386-018-0051-7
Raballo, A. (2018). Digital phenotyping: an overarching framework to capture our extended mental states. The Lancet. Psychiatry, 5(3), 194–195. https://doi.org/10.1016/S2215-0366(18)30054-3
Teo, J. X., Davila, S., Yang, C., Hii, A. A., Pua, C. J., Yap, J., … Lim, W. K. (2019). Digital phenotyping by consumer wearables identifies sleep-associated markers of cardiovascular disease risk and biological aging. Communications Biology, 2, 361. https://doi.org/10.1038/s42003-019-0605-1
Torous, J. et al. (2019). Digital phenotyping for the busy psychiatrist: clinical implications and relevance. Psychiatric Annals, 49(5), 196–201.
Vaidyam, A., Halamka, J., & Torous, J. (2019). Actionable digital phenotyping: a framework for the delivery of just-in-time and longitudinal interventions in clinical healthcare. MHealth, 5, 25. https://doi.org/10.21037/mhealth.2019.07.04