John Gideon, M.S.’s study identifies the necessity of early detection of suicidal ideation being the key to suicidal prevention. A promising approach to symptom monitoring is suicidal speech prediction, as speech can be passively collected and may indicate changes in risk. Since suicidal ideation is associated with emotion dysregulation, John Gideon’s research focuses on the detection of emotion from speech and its relation to suicide.
Utilizing mobile Ecological Momentary Assessment, this study introduces the Ecological Measurement of Affect, Speech, and Suicide (EMASS) dataset, which contains phone call recordings of individuals recently discharged from the hospital following admission for suicidal ideation or behavior, along with controls. Participants self-reported their emotion periodically throughout the study.
Directly identifying suicidal speech is difficult, as characteristics of speech can vary rapidly compared with suicidal thoughts. The study demonstrates how outside emotion data sets can be used to generate more relevant features, making his analysis possible. The study also uses emotion predictions to differentiate healthy controls from those with suicidal ideation, providing evidence for suicidal speech detection using emotion.
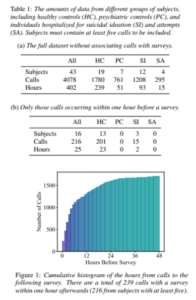
John Gideon, M.S.
Graduate Student Research Assistant at the University of Michigan
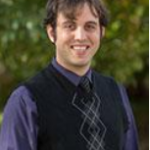
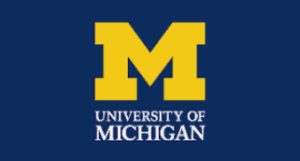